Your Idea Matters!
Pulvinar enim ac tortor nulla facilisi tristique facilisi
elementum sollicitudin eget lorem.
Local Business
Lorem ipsum dolor consectetur adipiscing elit eiusmod.
Online Store
Lorem ipsum dolor consectetur adipiscing elit eiusmod.
Blogging
Lorem ipsum dolor consectetur adipiscing elit eiusmod.
Portfolio
Lorem ipsum dolor consectetur adipiscing elit eiusmod.
Our Services
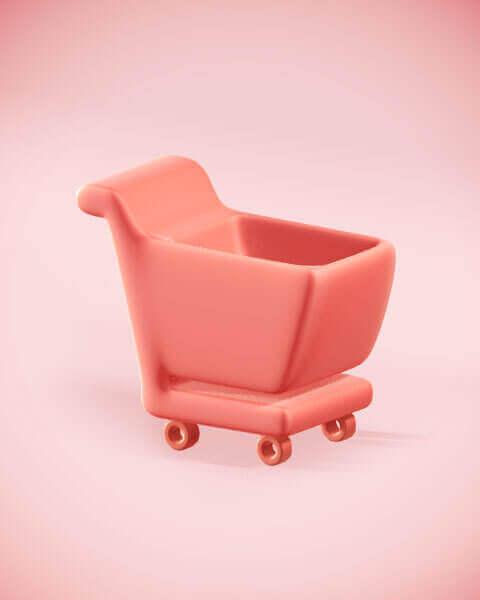
Branding Design
Sem quis erat nibh id neque tincidunt molestie convallis ut nibh vel, lorem consequat ullamcorper.
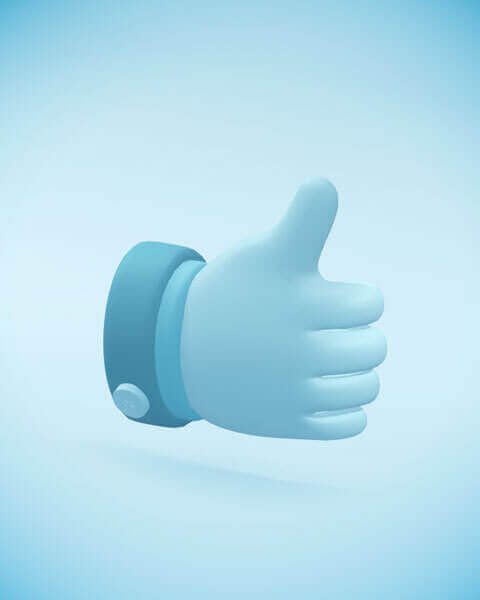
Graphic Design
Sem quis erat nibh id neque tincidunt molestie convallis ut nibh vel, lorem consequat ullamcorper.
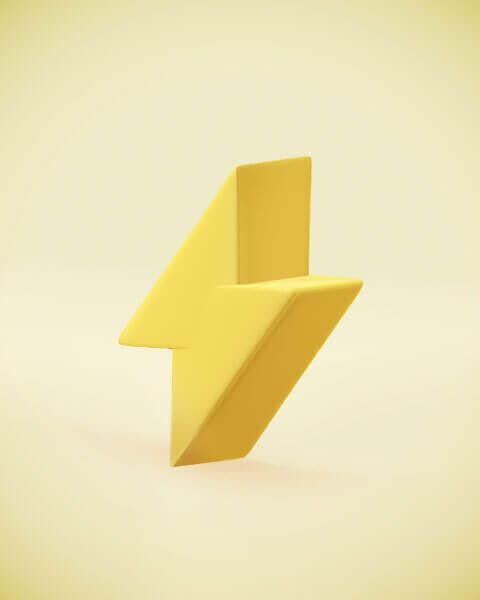
Web Development
Sem quis erat nibh id neque tincidunt molestie convallis ut nibh vel, lorem consequat ullamcorper.
We help teams build the business of their dreams
Et in risus egestas nec vitae odio ac nibh vestibulum volutpat aliquet aenean erat lobortis non.
Nibh egestas dictumst cursus est turpis quis tincidunt pulvinar maecenas eget massa vel, ante nam blandit egestas enim id quis sit maecenas id nunc tempus auctor orci, enim imperdiet proin nibh mattis.
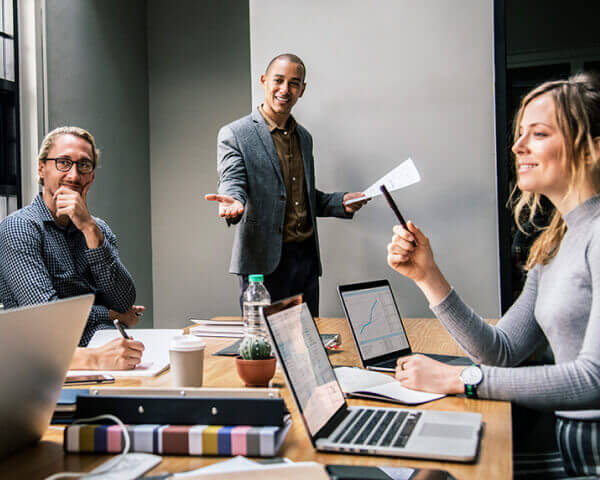

“The best part is that Astra comes with hundreds of professionally designed templates for just about every industry, makes it super easy for non-techy users to build a website.”
Wade Warren
Why Choose Us
Passionate
Tempor ullamcorper urna, est, lectus amet sit tempor pretium mi sed morbi cras posuere sit ultrices bibendum augue sit ornare.
Professional
Tempor ullamcorper urna, est, lectus amet sit tempor pretium mi sed morbi cras posuere sit ultrices bibendum augue sit ornare.
Support
Tempor ullamcorper urna, est, lectus amet sit tempor pretium mi sed morbi cras posuere sit ultrices bibendum augue sit ornare.